The ability of Numerical Weather Prediction (NWP) and climate models to accurately represent Arctic cyclones, tropopause polar vortices, and their interactions with cloud microphysics and surface heterogeneities will be assessed.
Different models are concidered for the French team’s studies:
- Global models:
- Regional models:
- AROME-Arctic3 4 5: The AROME-Arctic model with 2.5 km resolution centered over Svalbard and north of Norway used by the Norwegian Meteorological institute (MET Norway) for NWP, is a configuration of the ALADIN-HIRLAM cooperation.
- AROME6: A new version of AROME, the regional limited-are model operational at Météo-France on a domain over France, has been developed with the same domain over Svalbard as the AROME-Arctic model. This model differs from AROME-Arctic mainly by its cloud microphysic and turbulence schemes.
- Polar WRF7: Polar WRF is an optimized version of the state-of-the-art WRF mesoscale model for polar region.
- ICOLMDZ: This limited-area model use the new dynamical core DYNAMICO developped at Institut Pierre Simon Laplace with a icosahedric grid, and the physical parameterization of LMDZ.
The sensitivity to different parameters will be tested and analyzed (See table 1.1):
Model | Cloud Microphysical scheme available | Sensitivity to parameters | Sea ice | Resolution |
ARPEGE | Lopez (2002)8 | – liquid/ice partition function | GELATO1D, Bazile et al. (2020)9 | 5-10km |
LMDZ | Madeleine et al. (2020)10 | – liquid/ice partition function – ice snow fall speed | 50km in zoom | |
AROME-Arctic | Muller et al. (2017)11, Engdahl et al. 2020)12, Engdahl et al. (2022)13 | Batrak et al. (2018)14 | 2.5 km | |
AROME | – ICE3 (Pinty and Jabouille, 1998)15 – LIMA (Vié et al., 2016)16 | ICE3: – liquid/ice partition function | GELATO1D, Bazile et al. (2020) | 1.3 km |
Polar WRF | – Morrison et al (2009)17 – Milbrandt and Yau (2005)18 | 1 km, 5 km, 25 km | ||
ICOLMDZ | Madeleine et al. (2020) | 27.5 km |
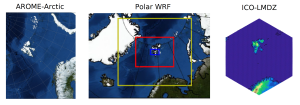
- Courtier P., C. Freydier, J. Geleyn, F. Rabier, M. Rochas, 1991. The ARPEGE project at Météo-France. In ECMWF Seminar Proceedings, Vol. II. ECWMF: Reading, UK, 193–231. ↩︎
- Hourdin, F., C. Rio, J.-Y. Grandpeix, J.-B. Madeleine, F. Cheruy, N. Rochetin, A. Jam, I. Musat, A. Idelkadi, L. Fairhead, M.-A. Foujols, L. Mellul, A.-K. Traore, J.-L. Dufresne, O. Boucher, M.-P. Lefebvre, E. Millour, E. Vignon, J. Jouhaud, F. Bint Diallo, F. Lott, G. Gastineau, A. Caubel, Y. Meurdesoif, J. Ghattas, 2020. LMDZ6A: The atmospheric component of the IPSL climate model with improved and better tuned physics. Journal of Advances in Modeling Earth Systems, 12, e2019MS001892. https://doi.org/10.1029/2019MS001892 ↩︎
- Müller, M., Y. Batrak, J. Kristiansen, M. A. Ø. Køltzow, G. Noer, and A. Korosov, 2017: Characteristics of a Convective-Scale Weather Forecasting System for the European Arctic. Monthly Weather Review, 145, 4771–4787, https://doi.org/10.1175/MWR-D-17-0194.1. ↩︎
- Køltzow, M., B. Casati, E. Bazile, T. Haiden and T. Valkonen, 2019: A NWP model inter-comparison of surface weather parameters in the European Arctic during the Year of Polar Prediction Special Observing Period Northern Hemisphere 1. Weather and Forecasting, 34, 959–983, https://doi.org/10.1175/WAF-D-19-0003.1. ↩︎
- Bengtsson, L., U. Andrae, T. Aspelien, Y. Batrak, J. Calvo, W. de Rooy, E. Gleeson, B. Hansen-Sass, M. Homleid, M. Hortal, K.-I. Ivarsson, G. Lenderink, S. Niemelä, K. P. Nielsen, J. Onvlee, L. Rontu, P. Samuelsson, D. Santos Muñoz, A. Subias, S. Tijm, V. Toll, X. Yang, and M. Ø. Køltzow, 2017: The HARMONIE–AROME Model Configuration in the ALADIN–HIRLAM NWP System. Monthly
Weather Review, 145, 1919–1935, https://doi.org/10.1175/MWR-D-16-0417.1. ↩︎ - Seity, Y., P. Brousseau, S. Malardel, G. Hello, P. Bénard, F. Bouttier, C. Lac, and V. Masson, 2011: The AROME-France Convective-Scale Operational Model. Monthly Weather Review, 139, 976–991, https://doi.org/10.1175/2010MWR3425.1. ↩︎
- Hines, K. M., and D. H. Bromwich, 2008: Development and Testing of Polar Weather Research and Forecasting (WRF) Model. Part I: Greenland Ice Sheet Meteorology. Monthly Weather Review, 136 (6), 1971–1989, https://doi.org/10.1175/2007MWR2112.1. ↩︎
- Lopez, P., 2002: Implementation and validation of a new prognostic large-scale cloud and precipitation scheme for climate and data-assimilation purposes. Quaterly Journal of the Royal Meteorological Society, 128: 229-257. https://doi.org/10.1256/00359000260498879 ↩︎
- Bazile, E., N. Azouz, A. Napoly, and C. Loo, 2020: Impact of the 1D sea-ice model GELATO in the global model ARPEGE. WCRP Rep. 12/2020, Research Activities in Earth System Modelling Rep. 50, 2 pp., http://bluebook.meteoinfo.ru/uploads/2020/docs/06_Bazile_Eric_sea_ice_model_in_ARPEGE.pdf. ↩︎
- Madeleine, J.-B., F. Hourdin, J.-Y. Grandpeix, C. Rio, J.-L. Dufresne, E. Vignon, et al. 2020: Improved representation of clouds in the atmospheric component LMDZ6A of the IPSL-CM6A Earth system model. Journal of Advances in Modeling Earth Systems, 12, e2020MS002046. https://doi.org/10.1029/2020MS002046 ↩︎
- Müller, M., M. Homleid, K.-I. Ivarsson, M. A. Ø. Køltzow, M. Lindskog, K. H. Midtbø, U. Andrae, T. Aspelien, L. Berggren, D. Bjørge, P. Dahlgren, J. Kristiansen, R. Randriamampianina, M. Ridal, O. Vignes, 2017: AROME-MetCoOp: A Nordic Convective-Scale Operational Weather Prediction Model. Weather Forecasting, 32, 609–627, https://doi.org/10.1175/WAF-D-16-0099.1. ↩︎
- Engdahl, B. J. K., B.E.K. Nygaard, V. Losnedal, G. Thompson, L. Bengtsson, 2020: Effects of the ICE-T microphysics scheme in HARMONIE-AROME on estimated ice loads on transmission lines, Cold Regions Science and Technology, Volume 179, 103139, https://doi.org/10.1016/j.coldregions.2020.103139. ↩︎
- Engdahl, B. J. K., T. Carlsen, M. Køltzow, and T. Storelvmo, 2022: The Ability of the ICE-T Microphysics Scheme in HARMONIE-AROME to Predict Aircraft Icing. Weather Forecasting, 37, 205–217, https://doi.org/10.1175/WAF-D-21-0104.1. ↩︎
- Batrak, Y., E. Kourzeneva, and M. Homleid, 2018: Implementation of a simple thermodynamic sea ice scheme, SICE version 1.0-38h1, within the ALADIN–HIRLAM numerical weather prediction system version 38h1. Geoscientific Model Development, 11, https://doi.org/10.5194/gmd-11-3347-2018. ↩︎
- Pinty, J.-P. and P. Jabouille, 1998: A mixed-phase cloud parameterization for use in mesoscale non-hydrostatic model: simulations of a squall line and of orographic precipitations. Proc. Conf. of Cloud Physics, Everett, WA, USA, Amer. Meteor. soc., Aug. 1999, 217 – 220. ↩︎
- Vié, B., J.-P. Pinty, S. Berthet and M.M. Leriche, 2016: LIMA (v1.0): A quasi two moment
microphysical scheme driven by a multimodal population of cloud condensation and ice freezing
nuclei, Geoscientific Model Development, 9, 567-586, https://doi.org/10.5194/gmd-9-567-2016 ↩︎ - Morrison, H., G. Thompson, and V. Tatarskii, 2009: Impact of Cloud Microphysics on the Development of Trailing Stratiform Precipitation in a Simulated Squall Line: Comparison of One- and Two-Moment Schemes. Monthly Weather Review, 137, 991–1007, https://doi.org/10.1175/2008MWR2556.1. ↩︎
- Milbrandt, J. A., and M. K. Yau, 2005: A Multimoment Bulk Microphysics Parameterization. Part I: Analysis of the Role of the Spectral Shape Parameter. Journal of Atmospheric Sciences, 62, 3051–3064, https://doi.org/10.1175/JAS3534.1. ↩︎